Natural Language Processing (NLP) is a subfield of artificial intelligence that focuses on enabling computers to understand, interpret, and generate human language in a way that is both meaningful and useful. NLP involves various tasks such as text classification, machine translation, sentiment analysis, and question-answering systems. Sentiment analysis, also known as opinion mining, is a specific NLP task that involves determining the sentiment or emotion expressed in a piece of text, typically categorizing it as positive, negative, or neutral. This can be useful for businesses to gauge customer feedback, track brand reputation, and improve customer service.
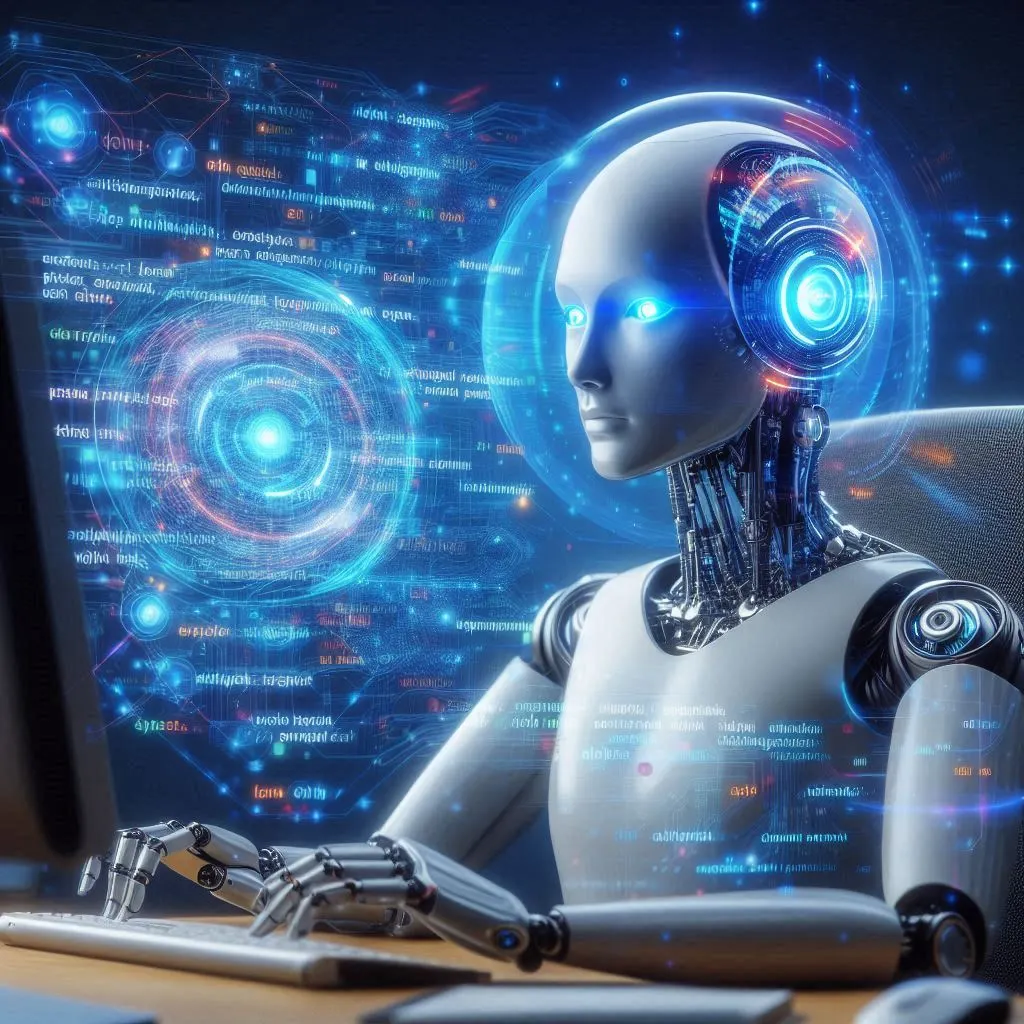
Table of Contents
- The Evolution of Natural Language Processing (NLP): A Comprehensive Overview
- Sentiment Analysis: Decoding Human Emotions through AI
- The Role of Natural Language Processing in Social Media Monitoring and Analysis
- The Future of Natural Language Processing : Challenges and Opportunities
- Real-life Applications of Natural Language Processing and Sentiment Analysis in Business and Marketing
- Q&A
The Evolution of Natural Language Processing (NLP): A Comprehensive Overview
Natural Language Processing (NLP) has come a long way since its inception, and has evolved into a powerful tool for understanding and analyzing human language. One of the most significant advancements in NLP is sentiment analysis, which has the potential to transform the way businesses interact with their customers and how individuals communicate with one another.
Sentiment analysis, also known as opinion mining, is the process of determining the sentiment or emotion behind a piece of text. This can be done through various techniques, such as machine learning algorithms, rule-based systems, and deep learning models. The goal of sentiment analysis is to identify the underlying sentiment of a text, whether it is positive, negative, or neutral.
The evolution of Natural Language Processing has led to the development of more sophisticated sentiment analysis techniques. Early approaches to sentiment analysis relied on simple keyword matching, where specific words or phrases were associated with a particular sentiment. For example, the word “great” might be associated with a positive sentiment, while the word “terrible” would be associated with a negative sentiment. While this approach was relatively straightforward, it was also limited in its ability to accurately capture the nuances of human language.
As Natural Language Processing has evolved, so too have the techniques used for sentiment analysis. One of the most significant advancements in this area is the use of machine learning algorithms. These algorithms can learn to identify patterns in text data that are indicative of a particular sentiment. For example, a machine learning algorithm might learn that the presence of certain words or phrases, such as “excellent” or “poor,” is indicative of a positive or negative sentiment, respectively.
Another important development in the field of Natural Language Processing is the use of deep learning models for sentiment analysis. Deep learning models, such as recurrent neural networks (RNNs) and convolutional neural networks (CNNs), are capable of learning complex patterns in text data that are difficult for traditional machine learning algorithms to capture. These models can also take into account the context in which words and phrases appear, which can help to improve the accuracy of sentiment analysis.
The use of Natural Language Processing and sentiment analysis has far-reaching implications for businesses and individuals alike. For businesses, sentiment analysis can provide valuable insights into customer opinions and preferences. By analyzing customer reviews, social media posts, and other forms of text data, businesses can gain a better understanding of their customers’ needs and preferences. This information can be used to inform product development, marketing strategies, and customer service initiatives.
For individuals, sentiment analysis can help to improve communication and understanding between people. By analyzing the sentiment behind text messages, emails, and other forms of communication, individuals can gain a better understanding of the emotions and intentions behind the words. This can help to improve relationships and foster greater understanding between people.
In conclusion, the evolution of Natural Language Processing (NLP) has led to significant advancements in sentiment analysis. By leveraging machine learning algorithms and deep learning models, NLP can accurately identify the sentiment behind text data, providing valuable insights for businesses and individuals alike. As Natural Language Processing continues to evolve, it is likely that sentiment analysis will become even more sophisticated, allowing for greater understanding and analysis of human language.
Sentiment Analysis: Decoding Human Emotions through AI
Natural Language Processing (NLP) and sentiment analysis have emerged as powerful tools in the field of artificial intelligence, enabling machines to understand and interpret human emotions and sentiments. These technologies have revolutionized the way businesses and organizations interact with their customers, providing valuable insights into consumer behavior and preferences.
Natural Language Processing (NLP) is a subfield of artificial intelligence that focuses on enabling computers to understand, interpret, and generate human language in a way that is both meaningful and useful. This involves the development of algorithms and models that can process and analyze large volumes of text data, extracting valuable information and insights. NLP has a wide range of applications, including machine translation, information extraction, and sentiment analysis.
Sentiment analysis, also known as opinion mining, is a specific application of NLP that involves the use of text analysis techniques to identify and extract subjective information in source materials. The primary goal of sentiment analysis is to determine the sentiment or emotion expressed in a piece of text, such as a review, comment, or social media post. This can be done by analyzing the words, phrases, and overall structure of the text, as well as the context in which it is used.
One of the key challenges in sentiment analysis is the ability to accurately identify and classify emotions. This requires a deep understanding of human language and the nuances of emotion expression. Machine learning algorithms, such as deep learning and support vector machines, are often used to train models that can recognize and classify emotions with a high degree of accuracy. These models are typically trained on large datasets of labeled text, which contain examples of different emotions and sentiment expressions.
Sentiment analysis has a wide range of applications in various industries. In the retail sector, for example, businesses can use sentiment analysis to monitor customer feedback and reviews, identifying trends and patterns in customer sentiment that can inform product development and marketing strategies. In the healthcare sector, sentiment analysis can be used to analyze patient feedback and identify areas for improvement in patient care and satisfaction.
In the realm of social media, sentiment analysis has become an essential tool for businesses and organizations looking to understand and engage with their audience. By analyzing the sentiment expressed in social media posts, businesses can gain valuable insights into consumer opinions and preferences, allowing them to tailor their marketing and communication strategies accordingly.
Despite the many benefits of sentiment analysis, there are also some limitations and challenges that need to be considered. One of the main challenges is the difficulty of accurately identifying and classifying emotions in text data, particularly when dealing with ambiguous or complex emotions. Additionally, the accuracy of sentiment analysis models can be affected by factors such as the quality of the training data, the complexity of the language used, and the presence of sarcasm or irony.
In conclusion, Natural Language Processing (NLP) and sentiment analysis have opened up new possibilities for businesses and organizations to understand and engage with their customers on a deeper level. By leveraging the power of AI and machine learning, these technologies can provide valuable insights into consumer behavior and preferences, enabling businesses to make more informed decisions and improve their overall customer experience. However, it is important to recognize the limitations and challenges associated with sentiment analysis, and to continue researching and developing new techniques and algorithms to improve its accuracy and effectiveness.
The Role of Natural Language Processing in Social Media Monitoring and Analysis
Natural Language Processing (NLP) has emerged as a powerful tool in the realm of social media monitoring and analysis. By leveraging the capabilities of Natural Language Processing , businesses can gain valuable insights into consumer sentiment, track brand mentions, and identify trends in real-time. In this article, we will delve into the role of Natural Language Processing in social media monitoring and analysis, focusing on its applications in sentiment analysis.
Sentiment analysis, also known as opinion mining, is a subfield of Natural Language Processing that involves the use of computational techniques to determine the sentiment or emotion behind a piece of text. This can be particularly useful in understanding consumer opinions and reactions to products, services, or events. In the context of social media, sentiment analysis can help businesses gauge public sentiment towards their brand, identify areas of improvement, and capitalize on positive feedback.
One of the key advantages of NLP in sentiment analysis is its ability to process large volumes of text data quickly and accurately. Social media platforms generate vast amounts of user-generated content every day, making manual analysis an impractical and time-consuming task. NLP algorithms can sift through this data, identify relevant content, and extract meaningful insights in a fraction of the time it would take a human analyst.
Moreover, NLP algorithms are capable of understanding the nuances of human language, such as sarcasm, irony, and idiomatic expressions. This enables them to accurately interpret the sentiment behind a piece of text, even when it may not be immediately apparent to a human reader. For example, a tweet that appears to be negative on the surface may actually be a joke or a form of praise in disguise. NLP algorithms can detect these subtleties and provide a more accurate representation of the sentiment.
In addition to sentiment analysis, Natural Language Processing can also be used for other social media monitoring tasks, such as brand mention tracking and trend identification. By analyzing the text of social media posts, NLP algorithms can identify when a brand is mentioned and categorize the mentions based on sentiment. This can help businesses monitor their online reputation and respond to customer feedback in a timely manner. Furthermore, NLP can be used to identify emerging trends and topics of conversation on social media, allowing businesses to stay ahead of the curve and capitalize on new opportunities.
Despite its many benefits, it is important to note that Natural Language Processing is not without its limitations. One of the main challenges is the difficulty of accurately interpreting sarcasm and other forms of irony, which can lead to misinterpretation of sentiment. Additionally, Natural Language Processing algorithms may struggle with understanding slang, abbreviations, and other forms of informal language commonly found on social media. As a result, businesses must be cautious in interpreting the results of Natural Language Processing analysis and consider them as a starting point for further investigation.
In conclusion, Natural Language Processing plays a crucial role in social media monitoring and analysis, particularly in sentiment analysis. By leveraging the power of Natural Language Processing , businesses can gain valuable insights into consumer sentiment, track brand mentions, and identify trends in real-time. However, it is important to recognize the limitations of NLP and use it as a tool to complement human analysis rather than replace it. As NLP technology continues to advance, it has the potential to revolutionize the way businesses interact with and understand their customers on social media.
The Future of Natural Language Processing : Challenges and Opportunities
Natural Language Processing (NLP) has come a long way since its inception, and one of the most promising areas of research in this field is sentiment analysis. Sentiment analysis, also known as opinion mining, is the process of determining the sentiment or emotion behind a piece of text. This can be particularly useful in understanding customer feedback, gauging public opinion on a particular topic, or even predicting stock market trends. In this article, we will explore the challenges and opportunities that lie ahead for NLP and sentiment analysis, as well as the potential impact on various industries.
One of the main challenges in Natural Language Processing and sentiment analysis is the ability to accurately interpret the context of a given text. This is especially true when dealing with sarcasm, irony, or other forms of figurative language. For example, a statement like “I love this movie” might be interpreted as positive sentiment, but if the speaker is being sarcastic, the sentiment could actually be negative. To overcome this challenge, NLP algorithms must be able to understand the nuances of human language and the context in which it is used.
Another challenge in Natural Language Processing and sentiment analysis is the need for large amounts of training data. In order to train a machine learning model to accurately classify sentiment, it requires a vast amount of labeled data. This can be time-consuming and expensive to obtain, especially when dealing with multiple languages or dialects. Additionally, the quality of the training data is crucial, as biased or inaccurate data can lead to poor model performance.
Despite these challenges, there are numerous opportunities for Natural Language Processing and sentiment analysis to make a significant impact in various industries. In the field of marketing, for example, sentiment analysis can be used to monitor customer feedback and identify areas for improvement. By understanding the sentiment behind customer reviews, businesses can make data-driven decisions to improve their products or services.
In the healthcare industry, sentiment analysis can be used to analyze patient feedback and identify trends in patient satisfaction. This information can be used to improve patient care and address any concerns that may arise. Additionally, sentiment analysis can be used to monitor social media for early detection of potential outbreaks or public health concerns.
In the finance sector, sentiment analysis can be used to predict stock market trends by analyzing news articles, social media posts, and other forms of text data. By understanding the sentiment behind financial news, investors can make more informed decisions about their investments.
In the field of education, sentiment analysis can be used to analyze student feedback and identify areas for improvement in teaching methods or curriculum. This information can be used to create a more engaging and effective learning experience for students.
In conclusion, NLP and sentiment analysis have the potential to revolutionize various industries by providing valuable insights into customer feedback, public opinion, and market trends. However, there are still challenges to overcome, such as understanding context and obtaining high-quality training data. As NLP continues to advance, we can expect to see even more opportunities for this technology to make a significant impact in the future.
Real-life Applications of Natural Language Processing and Sentiment Analysis in Business and Marketing
Natural Language Processing (NLP) and sentiment analysis have become increasingly important in the realm of business and marketing. These technologies enable businesses to better understand and respond to customer needs, preferences, and emotions. In this article, we will explore the real-life applications of NLP and sentiment analysis in business and marketing, and how they can be used to improve customer satisfaction, drive sales, and enhance brand reputation.
One of the primary applications of NLP and sentiment analysis in business is customer service. By analyzing customer feedback, reviews, and social media posts, businesses can gain valuable insights into customer satisfaction levels and identify areas for improvement. For example, a company might use NLP to analyze customer emails and identify common complaints or issues. This information can then be used to improve products or services, or to train customer service representatives to better address customer concerns.
Another important application of Natural Language Processing and sentiment analysis is in market research. By analyzing consumer opinions and preferences, businesses can identify trends and make informed decisions about product development, pricing, and marketing strategies. For example, a company might use NLP to analyze customer reviews of competing products to identify areas where their own product can be improved or differentiated. This information can then be used to develop new features or marketing campaigns that appeal to customers’ needs and preferences.
In addition to marketing, Natural Language Processing and sentiment analysis can be used to create targeted and personalized marketing campaigns. By analyzing customer data, businesses can identify customer preferences and emotions, and tailor marketing messages to resonate with individual customers. For example, a company might use NLP to analyze customer data and identify customers who are likely to be interested in a particular product or service. This information can then be used to create targeted marketing campaigns that are more likely to generate sales and conversions.
Furthermore, Natural Language Processing and sentiment analysis can be used to monitor and manage brand reputation. By analyzing customer feedback and social media posts, businesses can identify potential issues or negative sentiment that could harm their brand reputation. For example, a company might use NLP to analyze customer reviews and identify common complaints or negative sentiment. This information can then be used to address customer concerns and improve brand reputation.
In conclusion, Natural Language Processing (NLP) and sentiment analysis have a wide range of real-life applications in business and marketing. By analyzing customer feedback, reviews, and social media posts, businesses can gain valuable insights into customer satisfaction levels, preferences, and emotions. This information can be used to improve customer service, drive sales, and enhance brand reputation. As NLP and sentiment analysis technologies continue to advance, they will become even more powerful tools for businesses looking to better understand and respond to customer needs and preferences.
Q&A
1. What is Natural Language Processing (NLP)?
Natural Language Processing (NLP) is a subfield of artificial intelligence that focuses on enabling computers to understand, interpret, and generate human language in a way that is both meaningful and useful.
2. What is sentiment analysis?
Sentiment analysis, also known as opinion mining, is a subfield of Natural Language Processing that involves determining the sentiment or emotion expressed in a piece of text, such as a review, comment, or social media post. It typically classifies the sentiment as positive, negative, or neutral.
3. What are some common techniques used in NLP and sentiment analysis?
Some common techniques used in Natural Language Processing and sentiment analysis include:
– Tokenization: Breaking down text into individual words or tokens.
– Stopword removal: Removing common words that do not contribute to the meaning of the text.
– Stemming and lemmatization: Reducing words to their base or root form.
– Part-of-speech tagging: Identifying the grammatical role of each word in a sentence.
– Named entity recognition: Identifying and categorizing entities such as people, organizations, and locations.
– Word embeddings: Representing words as vectors in a high-dimensional space to capture semantic meaning.
– Machine learning and deep learning models: Training models to classify sentiment based on labeled training data.
4. What are some applications of Natural Language Processing and sentiment analysis?
NLP and sentiment analysis have a wide range of applications, including:
– Social media monitoring: Analyzing customer feedback and opinions on social media platforms.
– Customer support: Automating responses to customer inquiries and identifying common issues.
– Market research: Analyzing consumer opinions and trends to inform product development and marketing strategies.
– Sentiment-driven trading: Identifying market sentiment to inform investment decisions.
– Sentiment analysis in news articles: Understanding public opinion on current events and political issues.
5. What are some challenges in Natural Language Processing and sentiment analysis?
Some challenges in Natural Language Processing and sentiment analysis include:
– Ambiguity: Words can have multiple meanings, making it difficult for models to accurately interpret context.
– Sarcasm and irony: Detecting sarcasm and irony in text is challenging due to the subtle cues and nuances involved.
– Cultural and linguistic differences: Models need to be trained on diverse data to account for variations in language and cultural context.
– Noisy data: Text data can be noisy due to typos, abbreviations, and slang, which can make it difficult for models to accurately process and interpret the text.
Reference https://hbr.org/2022/04/the-power-of-natural-language-processing